Learning to Predict One or More Ranks in Ordinal Regression Tasks
Publication date:
Publisher version:
Descripción física:
Abstract:
We present nondeterministic hypotheses learned from an ordinal regression task. They try to predict the true rank for an entry, but when the classification is uncertain the hypotheses predict a set of consecutive ranks (an interval). The aim is to keep the set of ranks as small as possible, while still containing the true rank. The justification for learning such a hypothesis is based on a real world problem arisen in breeding beef cattle. After defining a family of loss functions inspired in Information Retrieval, we derive an algorithm for minimizing them. The algorithm is based on posterior probabilities of ranks given an entry. A couple of implementations are compared: one based on a multiclass SVM and other based on Gaussian processes designed to minimize the linear loss in ordinal regression tasks
We present nondeterministic hypotheses learned from an ordinal regression task. They try to predict the true rank for an entry, but when the classification is uncertain the hypotheses predict a set of consecutive ranks (an interval). The aim is to keep the set of ranks as small as possible, while still containing the true rank. The justification for learning such a hypothesis is based on a real world problem arisen in breeding beef cattle. After defining a family of loss functions inspired in Information Retrieval, we derive an algorithm for minimizing them. The algorithm is based on posterior probabilities of ranks given an entry. A couple of implementations are compared: one based on a multiclass SVM and other based on Gaussian processes designed to minimize the linear loss in ordinal regression tasks
Identificador local:
451
Collections
- Informática [803]
- Ponencias, Discursos y Conferencias [4062]
Files in this item
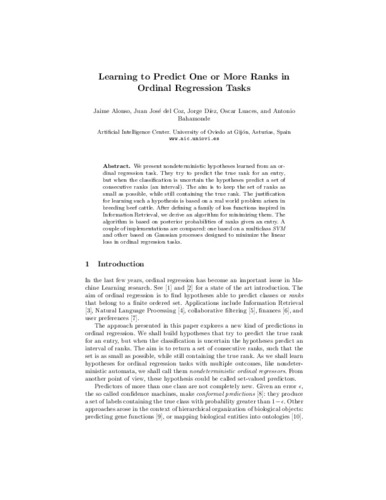