Aggregating independent and dependent models to learn multi-label classifiers
Publication date:
Editorial:
Springer
Publisher version:
Descripción física:
Abstract:
The aim of multi-label classi cation is to automatically obtain models able to tag objects with the labels that better describe them. Despite it could seem like any other classi cation task, it is widely known that exploiting the presence of certain correlations between labels helps to improve the classi cation performance. In other words, object descriptions are usually not enough to induce good models, also label information must be taken into account. This paper presents an aggregated approach that combines two groups of classi ers, one assuming independence between labels, and the other considering fully conditional dependence among them. The framework proposed here can be applied not only for multi-label classi cation, but also in multi-label ranking tasks. Experiments carried out over several datasets endorse the superiority of our approach with regard to other methods in terms of some evaluation measures, keeping competitiveness in terms of others
The aim of multi-label classi cation is to automatically obtain models able to tag objects with the labels that better describe them. Despite it could seem like any other classi cation task, it is widely known that exploiting the presence of certain correlations between labels helps to improve the classi cation performance. In other words, object descriptions are usually not enough to induce good models, also label information must be taken into account. This paper presents an aggregated approach that combines two groups of classi ers, one assuming independence between labels, and the other considering fully conditional dependence among them. The framework proposed here can be applied not only for multi-label classi cation, but also in multi-label ranking tasks. Experiments carried out over several datasets endorse the superiority of our approach with regard to other methods in terms of some evaluation measures, keeping competitiveness in terms of others
Description:
European Conference, ECML PKDD 2011, Athens, Greece, September 5-9, 2011
ISBN:
Identificador local:
20111143
Patrocinado por:
This research has been partially supported by Spanish Ministerio de Ciencia e Innovaci on (MICINN) grant TIN2008-06247
Collections
- Capítulos de libros [6507]
- Informática [872]
- Investigaciones y Documentos OpenAIRE [8365]
Files in this item
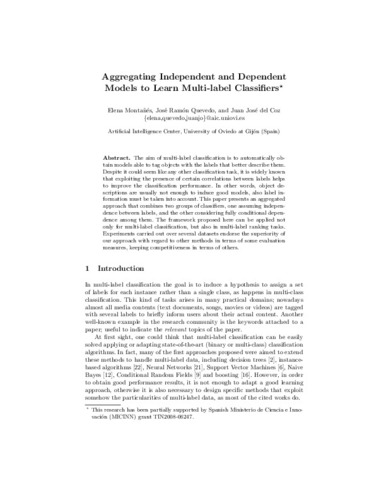