Learning data structure from classes: A case study applied to population genetics
Subject:
Clustering
Hierarchical classification
Pairwise classification
Publication date:
Editorial:
Elsevier
Publisher version:
Citación:
Abstract:
In most cases, the main goal of machine learning and data mining applications is to obtain good classifiers. However, final users, for instance researchers in other fields, sometimes prefer to infer new knowledge about their domain that may be useful to confirm or reject their hypotheses. This paper presents a learning method that works along these lines, in addition to reporting three interesting applications in the field of population genetics in which the aim is to discover relationships between species or breeds according to their genotypes. The proposed method has two steps: first it builds a hierarchical clustering of the set of classes and then a hierarchical classifier is learned. Both models can be analyzed by experts to extract useful information about their domain. In addition, we propose a new method for learning the hierarchical classifier. By means of a voting scheme employing pairwise binary models constrained by the hierarchical structure, the proposed classifier is computationally more efficient than previous approaches while improving on their performance
In most cases, the main goal of machine learning and data mining applications is to obtain good classifiers. However, final users, for instance researchers in other fields, sometimes prefer to infer new knowledge about their domain that may be useful to confirm or reject their hypotheses. This paper presents a learning method that works along these lines, in addition to reporting three interesting applications in the field of population genetics in which the aim is to discover relationships between species or breeds according to their genotypes. The proposed method has two steps: first it builds a hierarchical clustering of the set of classes and then a hierarchical classifier is learned. Both models can be analyzed by experts to extract useful information about their domain. In addition, we propose a new method for learning the hierarchical classifier. By means of a voting scheme employing pairwise binary models constrained by the hierarchical structure, the proposed classifier is computationally more efficient than previous approaches while improving on their performance
ISSN:
Identificador local:
20120237
Collections
- Artículos [36307]
- Informática [803]
Files in this item
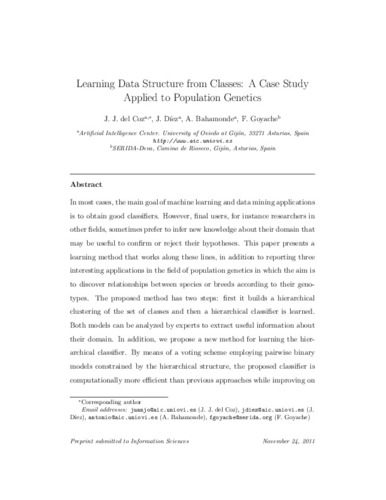