A semi-dependent decomposition approach to learn hierarchical classifiers
Subject:
Hierarchical classification
Multi-label learning
Structured output classification
Cost-sensitive learning
Support vector machines
Publication date:
Editorial:
Elsevier
Publisher version:
Citación:
Descripción física:
Abstract:
In hierarchical classification, classes are arranged in a hierarchy represented by a tree or a forest, and each example is labeled with a set of classes located on paths from roots to leaves or internal nodes. In other words, both multiple and partial paths are allowed. A straightforward approach to learn a hierarchical classifier, usually used as a baseline method, consists in learning one binary classifier for each node of the hierarchy; the hierarchical classifier is then obtained using a top-down evaluation procedure. The main drawback of this na¨ıve approach is that these binary classifiers are constructed independently, when it is clear that there are dependencies between them that are motivated by the hierarchy and the evaluation procedure employed. In this paper, we present a new decomposition method in which each node classifier is built taking into account other classifiers, its descendants, and the loss function used to measure the goodness of hierarchical classifiers. Following a bottom-up learning strategy, the idea is to optimize the loss function at every subtree assuming that all classifiers are known except the one at the root. Experimental results show that the proposed approach has accuracies comparable to state-of-the-art hierarchical algorithms and is better than the na¨ıve baseline method described above. Moreover, the benefits of our proposal include the possibility of parallel implementations, as well as the use of all available well-known techniques to tune binary classification SVMs
In hierarchical classification, classes are arranged in a hierarchy represented by a tree or a forest, and each example is labeled with a set of classes located on paths from roots to leaves or internal nodes. In other words, both multiple and partial paths are allowed. A straightforward approach to learn a hierarchical classifier, usually used as a baseline method, consists in learning one binary classifier for each node of the hierarchy; the hierarchical classifier is then obtained using a top-down evaluation procedure. The main drawback of this na¨ıve approach is that these binary classifiers are constructed independently, when it is clear that there are dependencies between them that are motivated by the hierarchy and the evaluation procedure employed. In this paper, we present a new decomposition method in which each node classifier is built taking into account other classifiers, its descendants, and the loss function used to measure the goodness of hierarchical classifiers. Following a bottom-up learning strategy, the idea is to optimize the loss function at every subtree assuming that all classifiers are known except the one at the root. Experimental results show that the proposed approach has accuracies comparable to state-of-the-art hierarchical algorithms and is better than the na¨ıve baseline method described above. Moreover, the benefits of our proposal include the possibility of parallel implementations, as well as the use of all available well-known techniques to tune binary classification SVMs
ISSN:
Identificador local:
20100287
Collections
- Artículos [37532]
- Informática [872]
Files in this item
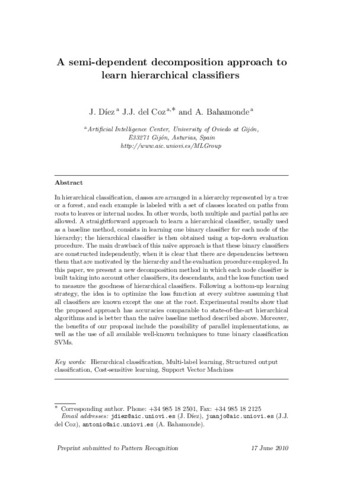