Multivariate Look-Up Table Based on N-Linear Interpolation for General Reflectarray Design
Subject:
Database
look-up table (LUT)
N-linear interpolation
machine learning
surrogate model
support vector regression (SVR)
crosspolar optimization
reflectarray antenna
Publication date:
Abstract:
We present a multivariate look-up table (LUT) based on N-linear interpolation for a fast general reflectarray design. In order to achieve an efficient implementation of the LUT, details are provided on its structure and a technique for a fast memory access of the reflection coefficients is described. A general formulation for the N-linear interpolation is given and then it is applied to a general reflectarray design procedure, including the improvement of the cross-polarization figures of merit. In order to benchmark the computational efficiency of the LUT, it is compared with other two tools, a method of moments based on local periodicity and surrogate models based on support vector regression. Results show that the LUT achieves a high computational efficiency while preserving a high degree of accuracy.
We present a multivariate look-up table (LUT) based on N-linear interpolation for a fast general reflectarray design. In order to achieve an efficient implementation of the LUT, details are provided on its structure and a technique for a fast memory access of the reflection coefficients is described. A general formulation for the N-linear interpolation is given and then it is applied to a general reflectarray design procedure, including the improvement of the cross-polarization figures of merit. In order to benchmark the computational efficiency of the LUT, it is compared with other two tools, a method of moments based on local periodicity and surrogate models based on support vector regression. Results show that the LUT achieves a high computational efficiency while preserving a high degree of accuracy.
Description:
Simposium Nacional de la Unión Científica Internacional de Radio, URSI (37º. 2022. Málaga, Spain)
Patrocinado por:
This work was supported in part by the Ministerio de Ciencia, Innovación y Universidades under project IJC2018-035696-I; by the Ministerio de Ciencia e Innovación and the Agencia Estatal de Investigación within project ENHANCE-5G (PID2020-114172RB-C21 / AEI / 10.13039/501100011033).
Collections
Files in this item
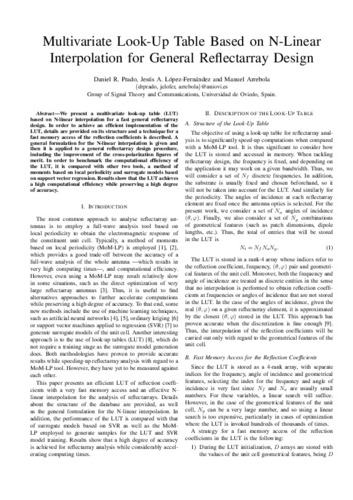