Why is quantification an interesting learning problem?
Autor(es) y otros:
Palabra(s) clave:
Sentiment analysis
Opinion mining
Quantification
Prevalence estimation
Fecha de publicación:
Editorial:
Springer
Versión del editor:
Citación:
Descripción física:
Resumen:
There are real applications that do not demand to classify or to make predictions about individual objects, but to estimate some magnitude about a group of them. For instance, one of these cases happens in sentiment analysis and opinion mining. Some applications require to classify opinions as positives or negatives, but there are also others, even more useful sometimes, that just need an estimation of which is the proportion of each class during a concrete period of time. “How many tweets about our new product were positive yesterday?” Practitioners should apply quantification algorithms to tackle this kind of problems, instead of just using off-the-shelf classification methods, because classifiers are suboptimal in the context of quantification tasks. Unfortunately, quantification learning is still relatively an under explored area in machine learning. The goal of this paper is to show that quantification learning is an interesting open problem. To support its benefits, we shall show an application to analyze Twitter comments in which even the most simple quantification methods outperform classification approaches
There are real applications that do not demand to classify or to make predictions about individual objects, but to estimate some magnitude about a group of them. For instance, one of these cases happens in sentiment analysis and opinion mining. Some applications require to classify opinions as positives or negatives, but there are also others, even more useful sometimes, that just need an estimation of which is the proportion of each class during a concrete period of time. “How many tweets about our new product were positive yesterday?” Practitioners should apply quantification algorithms to tackle this kind of problems, instead of just using off-the-shelf classification methods, because classifiers are suboptimal in the context of quantification tasks. Unfortunately, quantification learning is still relatively an under explored area in machine learning. The goal of this paper is to show that quantification learning is an interesting open problem. To support its benefits, we shall show an application to analyze Twitter comments in which even the most simple quantification methods outperform classification approaches
Patrocinado por:
This research has been funded by MINECO (the Spanish Ministerio de Econom´ıa y Competitividad) and FEDER (Fondo Europeo de Desarrollo Regional), grant TIN2015-65069-C2-2-R. Juan Jos´e del Coz is also supported by the Fulbright Commission and the Salvador de Madariaga Program, grant PRX15/00607. This paper has been written during the stay of Juan Jos´e del Coz at the University of Notre Dame
Colecciones
- Artículos [36139]
- Informática [789]
- Investigaciones y Documentos OpenAIRE [7870]
Ficheros en el ítem
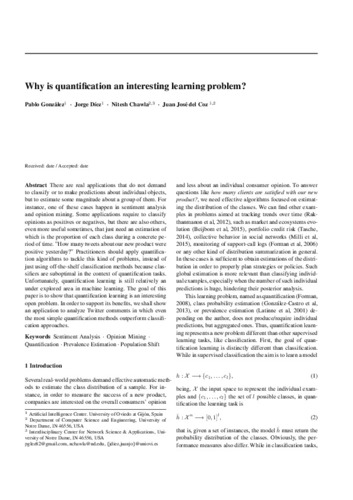