An overview of Inference Methods in Probabilistic Classifier Chains for Multi-label classification
Autor(es) y otros:
Fecha de publicación:
Editorial:
Wiley
Versión del editor:
Citación:
Descripción física:
Resumen:
This study presents a review of the recent advances in performing inference in probabilistic classifier chains for multilabel classification. The interest of performing such inference arises in an attempt of improving the performance of the approach based on greedy search (the well‐known CC method) and simultaneously reducing the computational cost of an exhaustive search (the well‐known PCC method). Unlike PCC and as CC, inference techniques do not explore all the possible solutions, but they increase the performance of CC, sometimes reaching the optimal solution in terms of subset 0/1 loss, as PCC does. The ε‐approximate algorithm, the method based on a beam search and Monte Carlo sampling are those techniques. An exhaustive set of experiments over a wide range of datasets are performed to analyze not only to which extent these techniques tend to produce optimal solutions, otherwise also to study their computational cost, both in terms of solutions explored and execution time. Only ε‐approximate algorithm with ε=.0 theoretically guarantees reaching an optimal solution in terms of subset 0/1 loss. However, the other algorithms provide solutions close to an optimal solution, despite the fact they do not guarantee to reach an optimal solution. The ε‐approximate algorithm is the most promising to balance the performance in terms of subset 0/1 loss against the number of solutions explored and execution time. The value of ε determines a degree to which one prefers to guarantee to reach an optimal solution at the expense of increasing the computational cost
This study presents a review of the recent advances in performing inference in probabilistic classifier chains for multilabel classification. The interest of performing such inference arises in an attempt of improving the performance of the approach based on greedy search (the well‐known CC method) and simultaneously reducing the computational cost of an exhaustive search (the well‐known PCC method). Unlike PCC and as CC, inference techniques do not explore all the possible solutions, but they increase the performance of CC, sometimes reaching the optimal solution in terms of subset 0/1 loss, as PCC does. The ε‐approximate algorithm, the method based on a beam search and Monte Carlo sampling are those techniques. An exhaustive set of experiments over a wide range of datasets are performed to analyze not only to which extent these techniques tend to produce optimal solutions, otherwise also to study their computational cost, both in terms of solutions explored and execution time. Only ε‐approximate algorithm with ε=.0 theoretically guarantees reaching an optimal solution in terms of subset 0/1 loss. However, the other algorithms provide solutions close to an optimal solution, despite the fact they do not guarantee to reach an optimal solution. The ε‐approximate algorithm is the most promising to balance the performance in terms of subset 0/1 loss against the number of solutions explored and execution time. The value of ε determines a degree to which one prefers to guarantee to reach an optimal solution at the expense of increasing the computational cost
ISSN:
DOI:
Patrocinado por:
This research has been supported by the Spanish Ministerio de Economía y Competitividad (grants TIN2011-23558, TIN2015-65069)
Colecciones
- Artículos [36410]
- Informática [810]
- Investigaciones y Documentos OpenAIRE [8059]
Ficheros en el ítem
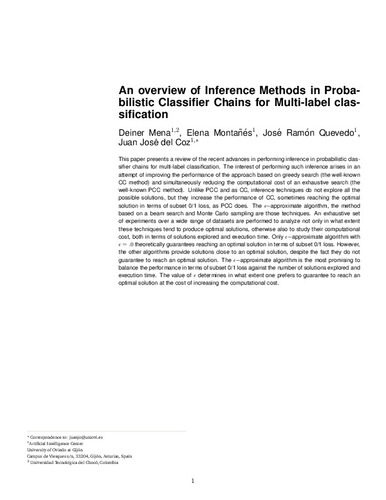