On the Problem of Error Propagation in classifier chains for multi-label classification
Autor(es) y otros:
Fecha de publicación:
Editorial:
Springer
Versión del editor:
Descripción física:
Resumen:
So-called classifier chains have recently been proposed as an appealing method for tackling the multi-label classification task. In this paper, we analyze the influence of a potential pitfall of the learning process, namely the discrepancy between the feature spaces used in training and testing: While true class labels are used as supplementary attributes for training the binary models along the chain, the same models need to rely on estimations of these labels when making a prediction. We provide first experimental results suggesting that the attribute noise thus created can affect the overall prediction performance of a classifier chain
So-called classifier chains have recently been proposed as an appealing method for tackling the multi-label classification task. In this paper, we analyze the influence of a potential pitfall of the learning process, namely the discrepancy between the feature spaces used in training and testing: While true class labels are used as supplementary attributes for training the binary models along the chain, the same models need to rely on estimations of these labels when making a prediction. We provide first experimental results suggesting that the attribute noise thus created can affect the overall prediction performance of a classifier chain
ISBN:
Patrocinado por:
This research has been supported by the Germany Research Foundation (DFG) and the Spanish Ministerio de Ciencia e Innovación (MICINN) under grant TIN2011-23558
Colecciones
- Capítulos de libros [6065]
- Informática [770]
- Investigaciones y Documentos OpenAIRE [7762]
Ficheros en el ítem
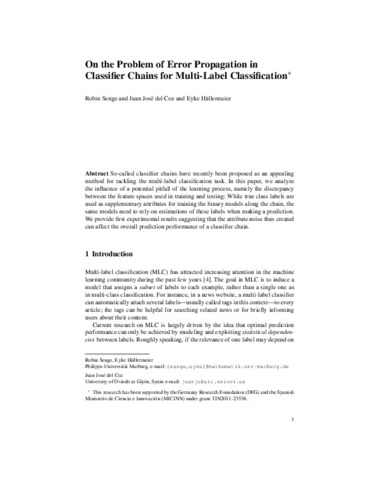