Peer assessment in MOOCs using preference learning via matrix factorization
Autor(es) y otros:
Fecha de publicación:
Resumen:
Evaluating in Massive Open Online Courses (MOOCs) is a difficult task because of the huge number of students involved in the courses. Peer grading is an effective method to cope with this problem, but something must be done to lessen the effect of the subjective evaluation. In this paper we present a matrix factorization approach able to learn from the order of the subset of exams evaluated by each grader. We tested this method on a data set provided by a real peer review process. By using a tailored graphical representation, the induced model could also allow the detection of peculiarities in the peer review process
Evaluating in Massive Open Online Courses (MOOCs) is a difficult task because of the huge number of students involved in the courses. Peer grading is an effective method to cope with this problem, but something must be done to lessen the effect of the subjective evaluation. In this paper we present a matrix factorization approach able to learn from the order of the subset of exams evaluated by each grader. We tested this method on a data set provided by a real peer review process. By using a tailored graphical representation, the induced model could also allow the detection of peculiarities in the peer review process
Colecciones
- Informática [789]
- Ponencias, Discursos y Conferencias [4044]
Ficheros en el ítem
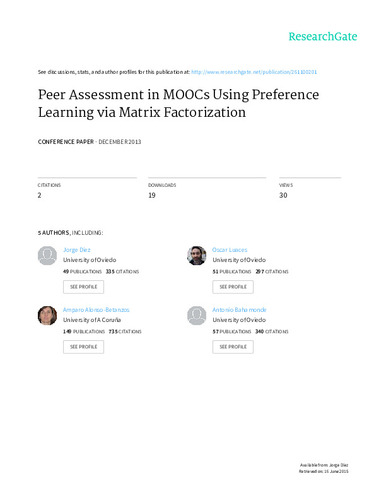