Improving Wearable-based Fall Detection with unsupervised learning
Palabra(s) clave:
Fall detection
Unsupervised learning
Clustering
One-class classifiers
Fecha de publicación:
Versión del editor:
Citación:
Descripción física:
Resumen:
Fall detection (FD) is a challenging task that has received the attention of the research community in the recent years. This study focuses on FD using data gathered from wearable devices with tri-axial accelerometers (3DACC), developing a solution centered in elderly people living autonomously. This research includes three different ways to improve a FD method: i) an analysis of the event detection stage, comparing several alternatives, ii) an evaluation of features to extract for each detected event and, iii) an appraisal of up to 6 different clustering scenarios to split the samples in subsets that might enhance the classification. For each clustering scenario, a specific classification stage is defined. The experimentation includes publicly available simulated fall data sets. Results show the guidelines for defining a more robust and efficient FD method for on-wrist 3DACC wearable devices.
Fall detection (FD) is a challenging task that has received the attention of the research community in the recent years. This study focuses on FD using data gathered from wearable devices with tri-axial accelerometers (3DACC), developing a solution centered in elderly people living autonomously. This research includes three different ways to improve a FD method: i) an analysis of the event detection stage, comparing several alternatives, ii) an evaluation of features to extract for each detected event and, iii) an appraisal of up to 6 different clustering scenarios to split the samples in subsets that might enhance the classification. For each clustering scenario, a specific classification stage is defined. The experimentation includes publicly available simulated fall data sets. Results show the guidelines for defining a more robust and efficient FD method for on-wrist 3DACC wearable devices.
ISSN:
Patrocinado por:
Spanish Ministry of Science and Innovation [MINECO-TIN2017-84804-R]; Asturias Regional Government [FCGRUPIN-IDI/2018/000226]; Instituto para la Competitividad Empresarial de Castilla y León [CCTT2/18/BU/0002]
Colecciones
Ficheros en el ítem
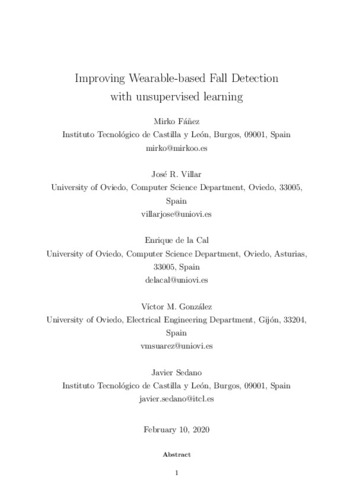