Automatic plankton quantification using deep features
Palabra(s) clave:
Abundance estimation
Deep learning
Convolutional neural networks
Phytoplankton
Fecha de publicación:
Editorial:
Oxford University Press
Versión del editor:
Citación:
Descripción física:
Resumen:
The study of marine plankton data is vital to monitor the health of the world’s oceans. In recent decades, automatic plankton recognition systems have proved useful to address the vast amount of data collected by specially engineered in situ digital imaging systems. At the beginning, these systems were developed and put into operation using traditional automatic classification techniques, which were fed with handdesigned local image descriptors (such as Fourier features), obtaining quite successful results. In the past few years, there have been many advances in the computer vision community with the rebirth of neural networks. In this paper, we leverage how descriptors computed using Convolutional Neural Networks (CNNs) trained with out-of-domain data are useful to replace hand-designed descriptors in the task of estimating the prevalence of each plankton class in a water sample. To achieve this goal, we have designed a broad set of experiments that show how effective these deep features are when working in combination with state-of-the-art quantification algorithms.
The study of marine plankton data is vital to monitor the health of the world’s oceans. In recent decades, automatic plankton recognition systems have proved useful to address the vast amount of data collected by specially engineered in situ digital imaging systems. At the beginning, these systems were developed and put into operation using traditional automatic classification techniques, which were fed with handdesigned local image descriptors (such as Fourier features), obtaining quite successful results. In the past few years, there have been many advances in the computer vision community with the rebirth of neural networks. In this paper, we leverage how descriptors computed using Convolutional Neural Networks (CNNs) trained with out-of-domain data are useful to replace hand-designed descriptors in the task of estimating the prevalence of each plankton class in a water sample. To achieve this goal, we have designed a broad set of experiments that show how effective these deep features are when working in combination with state-of-the-art quantification algorithms.
Patrocinado por:
Contributions from HMS and EEP were supported in part by the Simons Foundation, by the National Oceanic and Atmospheric Administration (NOAA) through the Cooperative Institute for the North Atlantic Region (CINAR) under Cooperative Agreement NA14OAR4320158, and by the National Science Foundation (NSF; Grants CCF-1539256, OCE-1655686)
Colecciones
- Artículos [36139]
- Informática [789]
- Investigaciones y Documentos OpenAIRE [7870]
Ficheros en el ítem
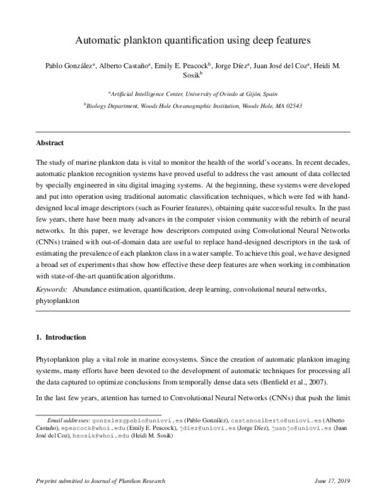