Functional testing techniques for new massive data processing paradigms
Otros títulos:
Técnicas de prueba funcionales para nuevos paradigmas de procesamiento masivo de datos
Autor(es) y otros:
Director(es):
Centro/Departamento/Otros:
Palabra(s) clave:
Big Data
MapReduce
Defectos funcionales
Fecha de publicación:
Descripción física:
Resumen:
Los programas Big Data son aquellos que analizan información utilizando nuevos modelos de procesamiento que superan las limitaciones de la tecnología tradicional en cuanto al volumen, velocidad y variedad de los datos procesados. Entre estos, se destaca MapReduce que permite procesar grandes cantidades de datos en una infraestructura distribuida que puede cambiar durante la ejecución debido a los frecuentes fallos en la infraestructura y las optimizaciones. El desarrollador sólo diseña el programa, mientras que la ejecución de su funcionalidad es gestionada por un sistema distribuido, tales como asignación de recursos y el mecanismo de tolerancia a fallos, entre otros. Como consecuencia, un programa puede comportarse diferente en cada ejecución porque se adapta automáticamente a los recursos que estén disponibles en cada momento. Esta ejecución no determinista dificulta las pruebas del software y la depuración, especialmente para aquellos programas MapReduce con un diseño complejo. A pesar de que tanto el rendimiento y la funcionalidad son importantes, la mayoría de investigación sobre la calidad de los programas MapReduce se centra en rendimiento. Por el contrario, hay pocos estudios sobre funcionalidad a pesar de que varias aplicaciones MapReduce fallan con regularidad debido a defectos funcionales. Probar y depurar estos defectos es importante, especialmente cuando los programas MapReduce realizan tareas críticas.= Big Data programs are those that analyse the information using new processing models to overcome the limitations of the traditional technology due the volume, velocity or variety of the data. Among them, MapReduce stands out by allowing for the processing of large data over a distributed infrastructure that can change during runtime due the frequent infrastructure failures and optimizations. The developer only designs the program, whereas the execution of its functionality is managed by a distributed system, such as the allocation of the resources and the fault tolerance mechanism, among others. As a consequence, a program can behave differently at each execution because it is automatically adapted to the resources available at each moment. This non-deterministic execution makes both software testing and debugging difficult, specially for those MapReduce programs with complex design. Despite both performance and functionality are important, the majority of the research about the quality of the MapReduce programs are focused on performance. In contrast, few research studies are about functionality although several MapReduce applications fail regularly due a functional fault. Testing and debugging these faults are important, specially when the MapReduce programs perform a critical task.
Los programas Big Data son aquellos que analizan información utilizando nuevos modelos de procesamiento que superan las limitaciones de la tecnología tradicional en cuanto al volumen, velocidad y variedad de los datos procesados. Entre estos, se destaca MapReduce que permite procesar grandes cantidades de datos en una infraestructura distribuida que puede cambiar durante la ejecución debido a los frecuentes fallos en la infraestructura y las optimizaciones. El desarrollador sólo diseña el programa, mientras que la ejecución de su funcionalidad es gestionada por un sistema distribuido, tales como asignación de recursos y el mecanismo de tolerancia a fallos, entre otros. Como consecuencia, un programa puede comportarse diferente en cada ejecución porque se adapta automáticamente a los recursos que estén disponibles en cada momento. Esta ejecución no determinista dificulta las pruebas del software y la depuración, especialmente para aquellos programas MapReduce con un diseño complejo. A pesar de que tanto el rendimiento y la funcionalidad son importantes, la mayoría de investigación sobre la calidad de los programas MapReduce se centra en rendimiento. Por el contrario, hay pocos estudios sobre funcionalidad a pesar de que varias aplicaciones MapReduce fallan con regularidad debido a defectos funcionales. Probar y depurar estos defectos es importante, especialmente cuando los programas MapReduce realizan tareas críticas.= Big Data programs are those that analyse the information using new processing models to overcome the limitations of the traditional technology due the volume, velocity or variety of the data. Among them, MapReduce stands out by allowing for the processing of large data over a distributed infrastructure that can change during runtime due the frequent infrastructure failures and optimizations. The developer only designs the program, whereas the execution of its functionality is managed by a distributed system, such as the allocation of the resources and the fault tolerance mechanism, among others. As a consequence, a program can behave differently at each execution because it is automatically adapted to the resources available at each moment. This non-deterministic execution makes both software testing and debugging difficult, specially for those MapReduce programs with complex design. Despite both performance and functionality are important, the majority of the research about the quality of the MapReduce programs are focused on performance. In contrast, few research studies are about functionality although several MapReduce applications fail regularly due a functional fault. Testing and debugging these faults are important, specially when the MapReduce programs perform a critical task.
Notas Locales:
DT(SE) 2019-079
Patrocinado por:
This work has been performed under the research projects TIN2013-46928-C3-1-R, funded by the Spanish Ministry of Economy and Competitiveness; GRUPIN14-007, funded by the Principality of Asturias (Spain); TIN2016-76956-C3-1-R, funded by the Spanish Ministry of Science and Technology; the Severo Ochoa pre-doctoral grant BP16215; and ERDF Funds.
Colecciones
- Investigaciones y Documentos OpenAIRE [8420]
- Tesis [7677]
- Tesis doctorales a texto completo [2172]
Ficheros en el ítem
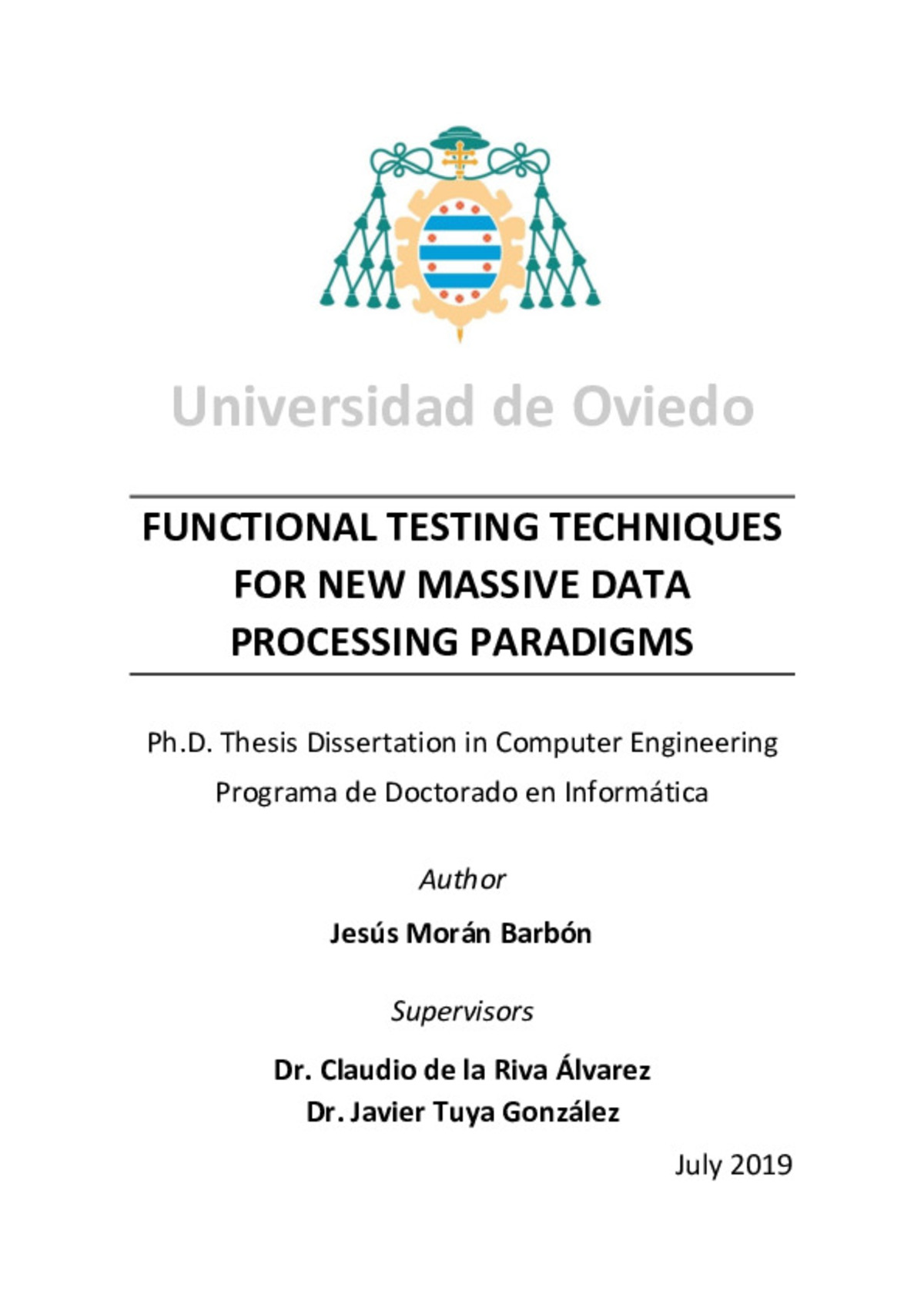