Optimizing novelty and diversity in recommendations
Publication date:
Editorial:
Springer
Publisher version:
Citación:
Descripción física:
Abstract:
The articles in the long tail are those that are not popular in some sense, but all together often represent a large proportion of the products covered by a recommender system. For companies, it is important to recommend these items that otherwise could be unknown to their customers. It is also interesting for users because knowing about these items might constitute a pleasant surprise. But long-tail items are not the only we might wish to recommend. Thus, some companies promote products on seasonal offers. It is a challenge to manage the preferences on items whose interaction with users is scarce. There is a trade-off between recommending items that users like and those belonging to a certain kind. We present a framework to address recommendations where the items will have a weight that quantifies our interest in recommending them in a broad sense. Then, we derive a factorization method that optimizes the award of the recommendations. To test the method, we present an exhaustive experimentation with a real-world dataset on digital news. We show that it is possible to improve dramatically the novelty (those items of special interest) and diversity of items with a tiny penalization in the accuracy
The articles in the long tail are those that are not popular in some sense, but all together often represent a large proportion of the products covered by a recommender system. For companies, it is important to recommend these items that otherwise could be unknown to their customers. It is also interesting for users because knowing about these items might constitute a pleasant surprise. But long-tail items are not the only we might wish to recommend. Thus, some companies promote products on seasonal offers. It is a challenge to manage the preferences on items whose interaction with users is scarce. There is a trade-off between recommending items that users like and those belonging to a certain kind. We present a framework to address recommendations where the items will have a weight that quantifies our interest in recommending them in a broad sense. Then, we derive a factorization method that optimizes the award of the recommendations. To test the method, we present an exhaustive experimentation with a real-world dataset on digital news. We show that it is possible to improve dramatically the novelty (those items of special interest) and diversity of items with a tiny penalization in the accuracy
Patrocinado por:
This work was funded by grants TIN2015-65069-C2-1-R and TIN2015-65069-C2-2-R from Ministerio de Economía y Competitividad
Collections
- Artículos [36307]
- Informática [803]
- Investigaciones y Documentos OpenAIRE [7936]
Files in this item
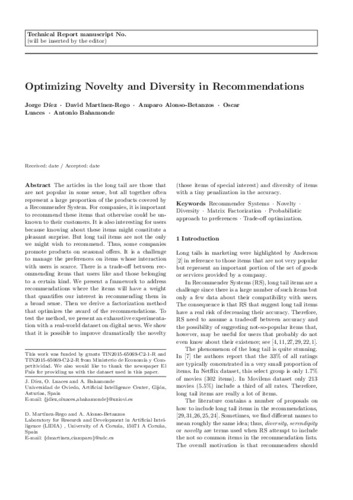