Content-based methods in peer assessment of open-response questions to grade students as authors and as graders
Autor(es) y otros:
Palabra(s) clave:
Peer assessment
Factorization
Preference learning
Grading graders
MOOCs
Fecha de publicación:
Editorial:
Elsevier
Versión del editor:
Citación:
Descripción física:
Resumen:
Massive Open Online Courses (MOOCs) use different types of assignments in order to evaluate student knowledge. Multiple-choice tests are particularly apt given the possibility for automatic assessment of large numbers of assignments. However, certain skills require open responses that cannot be assessed automatically yet their evaluation by instructors or teaching assistants is unfeasible given the large number of students. A potentially effective solution is peer assessment whereby students grade the answers of other students. However, to avoid bias due to inexperience, such grades must be filtered. We describe a factorization approach to grading, as a scalable method capable of dealing with very high volumes of data. Our method is also capable of representing open-response content using a vector space model of the answers. Since reliable peer assessment requires students to make coherent assessments, students can be motivated by their assessments reflecting not only their own answers but also their efforts as graders. The method described is able to tackle both these aspects simultaneously. Finally, for a real-world university setting in Spain, we compared grades obtained by our method and grades awarded by university instructors, with results indicating a notable improvement from using a content-based approach. There was no evidence that instructor grading would have led to more accurate grading outcomes than the assessment produced by our models
Massive Open Online Courses (MOOCs) use different types of assignments in order to evaluate student knowledge. Multiple-choice tests are particularly apt given the possibility for automatic assessment of large numbers of assignments. However, certain skills require open responses that cannot be assessed automatically yet their evaluation by instructors or teaching assistants is unfeasible given the large number of students. A potentially effective solution is peer assessment whereby students grade the answers of other students. However, to avoid bias due to inexperience, such grades must be filtered. We describe a factorization approach to grading, as a scalable method capable of dealing with very high volumes of data. Our method is also capable of representing open-response content using a vector space model of the answers. Since reliable peer assessment requires students to make coherent assessments, students can be motivated by their assessments reflecting not only their own answers but also their efforts as graders. The method described is able to tackle both these aspects simultaneously. Finally, for a real-world university setting in Spain, we compared grades obtained by our method and grades awarded by university instructors, with results indicating a notable improvement from using a content-based approach. There was no evidence that instructor grading would have led to more accurate grading outcomes than the assessment produced by our models
ISSN:
Patrocinado por:
This research was supported in part by the Spanish Ministerio de Economía y Competitividad (grants TIN2011-23558, TIN2012-37954, TIN2014-55894-C2- 2-R, TIN2015-65069-C2-1-R, TIN2015-65069-C2-2-R), the Junta de Andalucía (grant P12-TIC-1728) and the Xunta de Galicia (grant GRC2014/035), all, in turn, partially funded by FEDER. We would also like to thank the students from the University of A Coruña, Pablo de Olavide University and University of Oviedo who participated in this research
Colecciones
- Artículos [36307]
- Informática [803]
- Investigaciones y Documentos OpenAIRE [7936]
Ficheros en el ítem
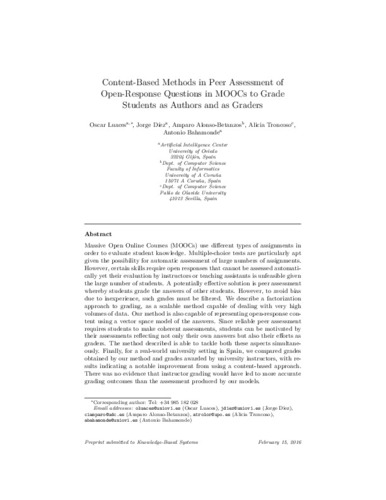