Using A* for inference in probabilistic classifier chains
Autor(es) y otros:
Fecha de publicación:
Editorial:
Association for the Advancement of Artificial Intelligence
Versión del editor:
Descripción física:
Resumen:
Probabilistic Classifiers Chains (PCC) offers interesting properties to solve multi-label classification tasks due to its ability to estimate the joint probability of the labels. However, PCC presents the major drawback of having a high computational cost in the inference process required to predict new samples. Lately, several approaches have been proposed to overcome this issue, including beam search and an -Approximate algorithm based on uniform-cost search. Surprisingly, the obvious possibility of using heuristic search has not been considered yet. This paper studies this alternative and proposes an admisible heuristic that, applied in combination with A* algorithm, guarantees, not only optimal predictions in terms of subset 0/1 loss, but also that it always explores less nodes than -Approximate algorithm. In the experiments reported, the number of nodes explored by our method is less than two times the number of labels for all datasets analyzed. But, the difference in explored nodes must be large enough to compensate the overhead of the heuristic in order to improve prediction time. Thus, our proposal may be a good choice for complex multi-label problems
Probabilistic Classifiers Chains (PCC) offers interesting properties to solve multi-label classification tasks due to its ability to estimate the joint probability of the labels. However, PCC presents the major drawback of having a high computational cost in the inference process required to predict new samples. Lately, several approaches have been proposed to overcome this issue, including beam search and an -Approximate algorithm based on uniform-cost search. Surprisingly, the obvious possibility of using heuristic search has not been considered yet. This paper studies this alternative and proposes an admisible heuristic that, applied in combination with A* algorithm, guarantees, not only optimal predictions in terms of subset 0/1 loss, but also that it always explores less nodes than -Approximate algorithm. In the experiments reported, the number of nodes explored by our method is less than two times the number of labels for all datasets analyzed. But, the difference in explored nodes must be large enough to compensate the overhead of the heuristic in order to improve prediction time. Thus, our proposal may be a good choice for complex multi-label problems
Descripción:
IJCAI-15, Buenos Aires, Argentina, 25–31 de julio de 2015
ISBN:
Patrocinado por:
Research supported by MINECO, grant TIN2011-23558
Colecciones
- Capítulos de libros [5888]
- Informática [758]
- Investigaciones y Documentos OpenAIRE [7560]
Ficheros en el ítem
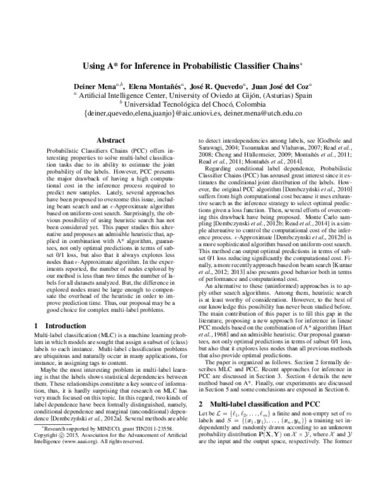