Adapting Decision DAGs for Multipartite Ranking
Autor(es) y otros:
Fecha de publicación:
Editorial:
Springer
Versión del editor:
Descripción física:
Resumen:
Multipartite ranking is a special kind of ranking for problems in which classes exhibit an order. Many applications require its use, for instance, granting loans in a bank, reviewing papers in a conference or just grading exercises in an education environment. Several methods have been proposed for this purpose. The simplest ones resort to regression schemes with a pre- and post-process of the classes, what makes them barely useful. Other alternatives make use of class order information or they perform a pairwise classi cation together with an aggregation function. In this paper we present and discuss two methods based on building a Decision Directed Acyclic Graph (DDAG). Their performance is evaluated over a set of ordinal benchmark data sets according to the C-Index measure. Both yield competitive results with regard to stateof- the-art methods, specially the one based on a probabilistic approach, called PR-DDAG
Multipartite ranking is a special kind of ranking for problems in which classes exhibit an order. Many applications require its use, for instance, granting loans in a bank, reviewing papers in a conference or just grading exercises in an education environment. Several methods have been proposed for this purpose. The simplest ones resort to regression schemes with a pre- and post-process of the classes, what makes them barely useful. Other alternatives make use of class order information or they perform a pairwise classi cation together with an aggregation function. In this paper we present and discuss two methods based on building a Decision Directed Acyclic Graph (DDAG). Their performance is evaluated over a set of ordinal benchmark data sets according to the C-Index measure. Both yield competitive results with regard to stateof- the-art methods, specially the one based on a probabilistic approach, called PR-DDAG
Descripción:
European Conference, ECML PKDD 2010, Barcelona, Spain, September 20-24, 2010
ISBN:
Patrocinado por:
This research has been partially supported by Spanish Ministerio de Ciencia e Innovaci on (MICINN) grants TIN2007-61273 and TIN2008-06247 and by FICYT, Asturias, Spain, under grant IB09-059-C2
Colecciones
- Capítulos de libros [6414]
- Informática [835]
- Investigaciones y Documentos OpenAIRE [8175]
Ficheros en el ítem
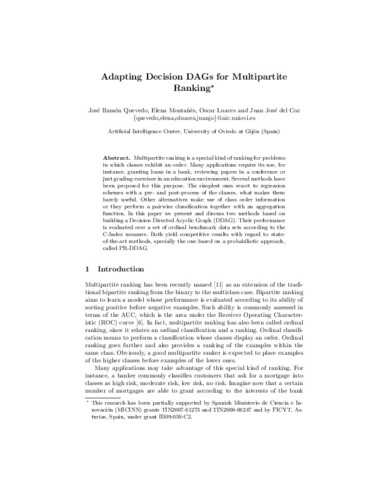