Prediction Model for Pollutants with Onboard Diagnostic Sensors in Vehicles
Autor(es) y otros:
Palabra(s) clave:
Sensors
Vehicles
Fecha de publicación:
Versión del editor:
Citación:
Descripción física:
Resumen:
In this work, a prediction model is developed to illustrate the relationship between the internal parameters of a vehicle and its emissions. Vehicles emit various hazardous pollutants and understanding the influence of in-vehicle parameters is key to reducing their environmental impact. The values of the internal parameters were collected through the On-Board Diagnostics port, while the values of the emissions were measured from the exhaust pipe using Arduino sensors. The observed values were then matched based on the timestamps received from both sources and fit with both linear and polynomial regressions to accurately model the relationship between the internal parameters and pollutants. These models can then be used to estimate vehicle emissions based on the in- vehicle parameters, including vehicle speed, relative throttle position, and engine revolutions per minute. A wide majority of the relationships between various in- vehicle parameters and emissions show no observable correlation. There are observable correlations between carbon dioxide emissions and vehicle speed, as well as carbon dioxide emissions and engine revolutions per minute. These relationships were modelled using linear and polynomial regression with a resulting adjusted R-squared value of approximately 0.1
In this work, a prediction model is developed to illustrate the relationship between the internal parameters of a vehicle and its emissions. Vehicles emit various hazardous pollutants and understanding the influence of in-vehicle parameters is key to reducing their environmental impact. The values of the internal parameters were collected through the On-Board Diagnostics port, while the values of the emissions were measured from the exhaust pipe using Arduino sensors. The observed values were then matched based on the timestamps received from both sources and fit with both linear and polynomial regressions to accurately model the relationship between the internal parameters and pollutants. These models can then be used to estimate vehicle emissions based on the in- vehicle parameters, including vehicle speed, relative throttle position, and engine revolutions per minute. A wide majority of the relationships between various in- vehicle parameters and emissions show no observable correlation. There are observable correlations between carbon dioxide emissions and vehicle speed, as well as carbon dioxide emissions and engine revolutions per minute. These relationships were modelled using linear and polynomial regression with a resulting adjusted R-squared value of approximately 0.1
ISSN:
Colecciones
- Artículos [37540]
- Informática [875]
Ficheros en el ítem
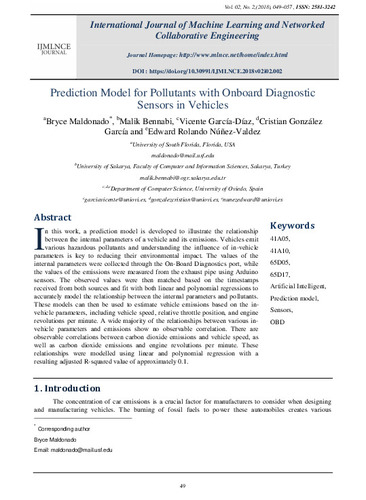