Stochastic Navigation in Smart Cities
Palabra(s) clave:
electric vehicle routing; charging stations; bio-inspired algorithm; stochastic process; smart cities
Fecha de publicación:
Versión del editor:
Citación:
Descripción física:
Resumen:
In this work we show how a simple model based on chemical signaling can reduce the exploration times in urban environments. The problem is relevant for smart city navigation where electric vehicles try to find recharging stations with unknown locations. To this end we have adapted the classical ant foraging swarm algorithm to urban morphologies. A perturbed Markov chain model is shown to qualitatively reproduce the observed behaviour. This consists of perturbing the lattice random walk with a set of perturbing sources. As the number of sources increases the exploration times decrease consistently with the swarm algorithm. This model provides a better understanding of underlying process dynamics. An experimental campaign with real prototypes provided experimental validation of our models. This enables us to extrapolate conclusions to optimize electric vehicle routing in real city topologies.
In this work we show how a simple model based on chemical signaling can reduce the exploration times in urban environments. The problem is relevant for smart city navigation where electric vehicles try to find recharging stations with unknown locations. To this end we have adapted the classical ant foraging swarm algorithm to urban morphologies. A perturbed Markov chain model is shown to qualitatively reproduce the observed behaviour. This consists of perturbing the lattice random walk with a set of perturbing sources. As the number of sources increases the exploration times decrease consistently with the swarm algorithm. This model provides a better understanding of underlying process dynamics. An experimental campaign with real prototypes provided experimental validation of our models. This enables us to extrapolate conclusions to optimize electric vehicle routing in real city topologies.
ISSN:
Colecciones
Ficheros en el ítem
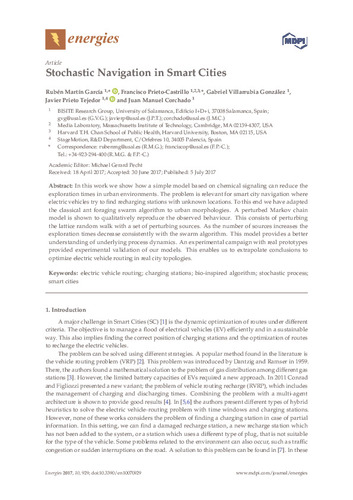