User-centered fall detection using supervised, on-line learning and transfer learning
Palabra(s) clave:
Fall detection
Smart wearables
Applied Machine Learning
Fecha de publicación:
Versión del editor:
Citación:
Descripción física:
Resumen:
There is no need to explain the relevance of fall detection (FD) in the elderly population: the faster they receive help, the higher the probabilities of recovery. FD has been widely studied, and a number of solutions have been proposed in the literature, including commercial products with well-known trademarks. Basically, the majority of the solutions include an Event Detection method followed by a feature extraction block as the inputs to a classifier that labels the sample as Fall or as Not Fall. Nevertheless, the proposals always rely on user feedback because of the relatively high percentage of false positives. This study proposes two supervised user-centered solutions for FD which are evaluated using two publicly available data sets that include simulated falls with the sensory system placed on a wrist. Instead of focusing on learning a generalized model, in this study we focus on learning specific models for each user. To overcome the lack of data from the Fall class, an on-line learning method to group the events arising from the activities of daily life and the consequent analysis of how fall events can then be detected is included. In addition, a transfer learning stage produces a priori knowledge from previous experiences that can be introduced into the on-line learning classifier in order to enhance its performance. Complete experimentation is carried out on two publicly available data sets, analyzing the performance of the supervised classifiers, and the performance of the whole detection system composed of the fall detection plus the classifier. In the case of on-line learning, only the results for the classifier, either with or without transfer learning, are included. The results suggest that FD user-centered solutions have the capacity to adapt to the information of a specific user, mostly when using both on-line learning and transfer learning. Nevertheless, the transfer learning stage needs refinements in order to avoid the complexity that this might introduce.
There is no need to explain the relevance of fall detection (FD) in the elderly population: the faster they receive help, the higher the probabilities of recovery. FD has been widely studied, and a number of solutions have been proposed in the literature, including commercial products with well-known trademarks. Basically, the majority of the solutions include an Event Detection method followed by a feature extraction block as the inputs to a classifier that labels the sample as Fall or as Not Fall. Nevertheless, the proposals always rely on user feedback because of the relatively high percentage of false positives. This study proposes two supervised user-centered solutions for FD which are evaluated using two publicly available data sets that include simulated falls with the sensory system placed on a wrist. Instead of focusing on learning a generalized model, in this study we focus on learning specific models for each user. To overcome the lack of data from the Fall class, an on-line learning method to group the events arising from the activities of daily life and the consequent analysis of how fall events can then be detected is included. In addition, a transfer learning stage produces a priori knowledge from previous experiences that can be introduced into the on-line learning classifier in order to enhance its performance. Complete experimentation is carried out on two publicly available data sets, analyzing the performance of the supervised classifiers, and the performance of the whole detection system composed of the fall detection plus the classifier. In the case of on-line learning, only the results for the classifier, either with or without transfer learning, are included. The results suggest that FD user-centered solutions have the capacity to adapt to the information of a specific user, mostly when using both on-line learning and transfer learning. Nevertheless, the transfer learning stage needs refinements in order to avoid the complexity that this might introduce.
ISSN:
Patrocinado por:
This research has been funded by the Spanish Ministry of Science and Innovation, under project MINECO-TIN2017-84804-R.
Colecciones
- Artículos [37354]
- Informática [865]
- Investigaciones y Documentos OpenAIRE [8230]
Ficheros en el ítem
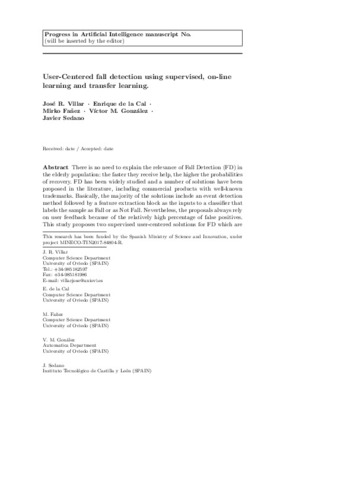