Fast and Accurate Lung Tumor Spotting and Segmentation for Boundary Delineation on CT Slices In A Coarse-To-Fine Framework
Autor(es) y otros:
Palabra(s) clave:
Boundary delineation
Lung tumor segmentation
Fully convolutional neural networks
Fecha de publicación:
Editorial:
Springer
Versión del editor:
Descripción física:
Resumen:
Label noise and class imbalance are two of the critical challenges when training image-based deep neural networks, especially in the biomedical image processing domain. Our work focuses on how to address the two challenges effectively and accurately in the task of lesion segmentation from biomedical/medical images. To address the pixel-level label noise problem, we propose an advanced transfer training and learning approach with a detailed DICOM pre-processing method. To address the tumor/non-tumor class imbalance problem, we exploit a self-adaptive fully convolutional neural network with an automated weight distribution mechanism to spot the Radiomics lung tumor regions accurately. Furthermore, an improved conditional random field method is employed to obtain sophisticated lung tumor contour delineation and segmentation. Finally, our approach has been evaluated using several well-known evaluation metrics on the Lung Tumor segmentation dataset used in the 2018 IEEE VIP-CUP Challenge. Experimental results show that our weakly supervised learning algorithm outperforms other deep models and state-of-the-art approaches
Label noise and class imbalance are two of the critical challenges when training image-based deep neural networks, especially in the biomedical image processing domain. Our work focuses on how to address the two challenges effectively and accurately in the task of lesion segmentation from biomedical/medical images. To address the pixel-level label noise problem, we propose an advanced transfer training and learning approach with a detailed DICOM pre-processing method. To address the tumor/non-tumor class imbalance problem, we exploit a self-adaptive fully convolutional neural network with an automated weight distribution mechanism to spot the Radiomics lung tumor regions accurately. Furthermore, an improved conditional random field method is employed to obtain sophisticated lung tumor contour delineation and segmentation. Finally, our approach has been evaluated using several well-known evaluation metrics on the Lung Tumor segmentation dataset used in the 2018 IEEE VIP-CUP Challenge. Experimental results show that our weakly supervised learning algorithm outperforms other deep models and state-of-the-art approaches
ISBN:
Colecciones
- Capítulos de libros [6237]
- Informática [806]
Ficheros en el ítem
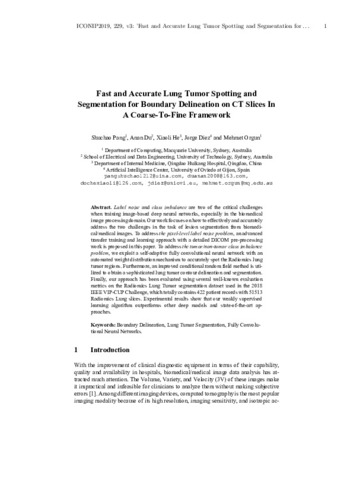