A factorization approach to evaluate open-response assignments in MOOCs using preference learning on peer assessments
Autor(es) y otros:
Palabra(s) clave:
Peer Grading
Factorization
MOOCs
Fecha de publicación:
Versión del editor:
Citación:
Descripción física:
Resumen:
Evaluating open-response assignments in Massive Open Online Courses is a difficult task because of the huge number of students involved. Peer grading is an effective method to address this problem. There are two basic approaches in the literature: cardinal and ordinal. The first case uses grades assigned by student-graders to a set of assignments of other colleagues. In the ordinal approach, the raw materials used by grading systems are the relative orders that graders appreciate in the assignments that they evaluate. In this paper we present a factorization method that seeks a trade-off between cardinal and ordinal approaches. The algorithm learns from preference judgments to avoid the subjectivity of the numeric grades. But in addition to preferences expressed by student-graders, we include other preferences: those induced from assignments with significantly different average grades. The paper includes a report of the results obtained using this approach in a real world dataset collected in 3 Universities of Spain, A Coruña, Pablo de Olavide at Sevilla, and Oviedo at Gijón. Additionally, we studied the sensitivity of the method with respect to the number of assignments graded by each student. Our method achieves similar or better scores than staff instructors when we measure the discrepancies with other instructor’s grades
Evaluating open-response assignments in Massive Open Online Courses is a difficult task because of the huge number of students involved. Peer grading is an effective method to address this problem. There are two basic approaches in the literature: cardinal and ordinal. The first case uses grades assigned by student-graders to a set of assignments of other colleagues. In the ordinal approach, the raw materials used by grading systems are the relative orders that graders appreciate in the assignments that they evaluate. In this paper we present a factorization method that seeks a trade-off between cardinal and ordinal approaches. The algorithm learns from preference judgments to avoid the subjectivity of the numeric grades. But in addition to preferences expressed by student-graders, we include other preferences: those induced from assignments with significantly different average grades. The paper includes a report of the results obtained using this approach in a real world dataset collected in 3 Universities of Spain, A Coruña, Pablo de Olavide at Sevilla, and Oviedo at Gijón. Additionally, we studied the sensitivity of the method with respect to the number of assignments graded by each student. Our method achieves similar or better scores than staff instructors when we measure the discrepancies with other instructor’s grades
Patrocinado por:
MINECO (Ministerio de Economia y Competitividad, Spain) [TIN2011-23558, TIN2012-37954, TIN2011-28956-C02]; FEDER funds
Colecciones
- Artículos [37540]
- Informática [875]
Ficheros en el ítem
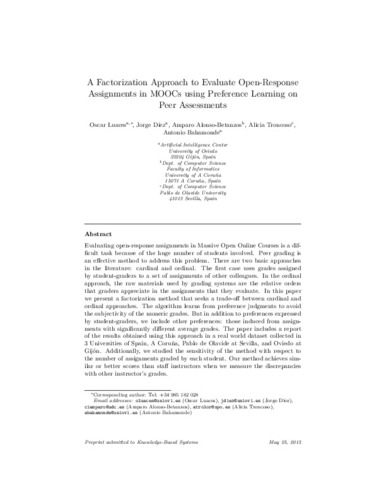